From 31st March to 4th April 2025, the Chemistry and Materials Machine Learning School (CaMML) brought together a dynamic group of students at STFC Daresbury, building foundational and advanced skills in machine learning for atomistic and molecular simulations.
This hands-on training was delivered by AIchemy in partnership with STFC and PSDI. Following the success of the 2023 Machine Learning for Atomistic Modelling Autumn School, this year’s course focused on PhD students with coding experience who are new to machine learning, particularly those in materials and molecular simulation fields.
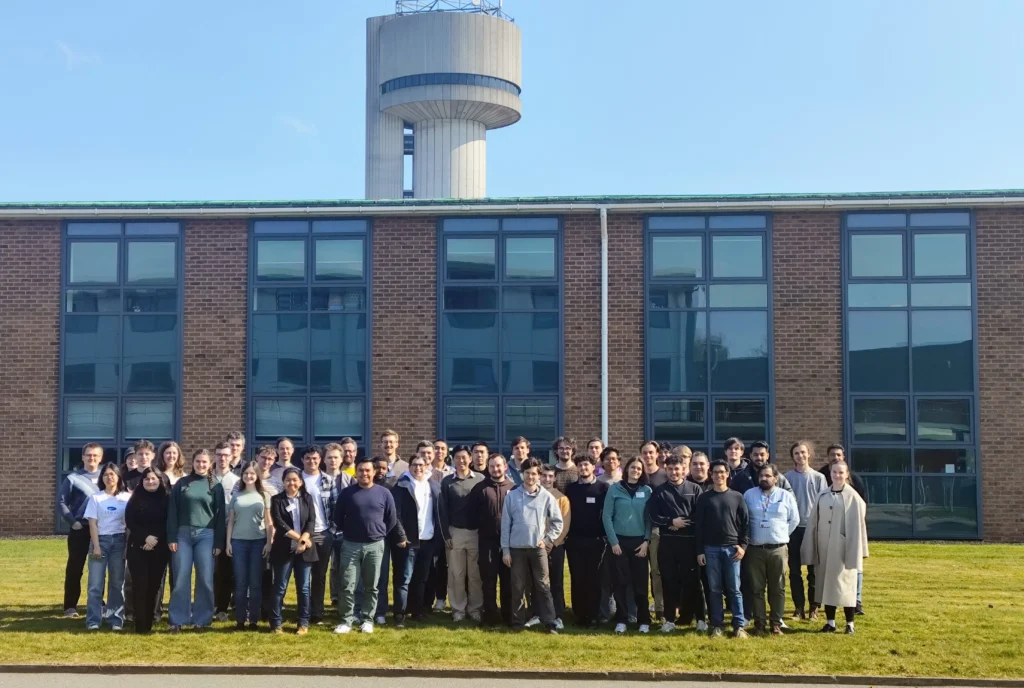
Participants gained practical skills and theoretical insights through expert-led talks and tutorials. Topics covered included:
- Intro to Machine Learning – Professor Reinhard Maurer
- Unsupervised ML & Graph Neural Networks – Dr. Alex Ganose
- Neural Networks & Generative Models – Dr. Keith Butler
- Machine Learning Interatomic Potentials – Dr. Ioan Magdau
- Research Seminars – Professor Kim Jelfs (Imperial College London) and Dr. Nong Artrith (University of Utrecht)
Beyond the technical training, attendees took part in poster sessions, networking events and socials, fostering a collaborative and interdisciplinary community.
🏆 Poster Prize Winners
- Simone Vari (Politecnico di Milano, Italy)
- Sophie Bennett (University of Southampton, UK)
- Gustavo Chaparro (Imperial College London, UK)
📝 Blog Content Winners
- Graph Neural Network – CC-BY by Timothée Jamin and Ahmed Ismail
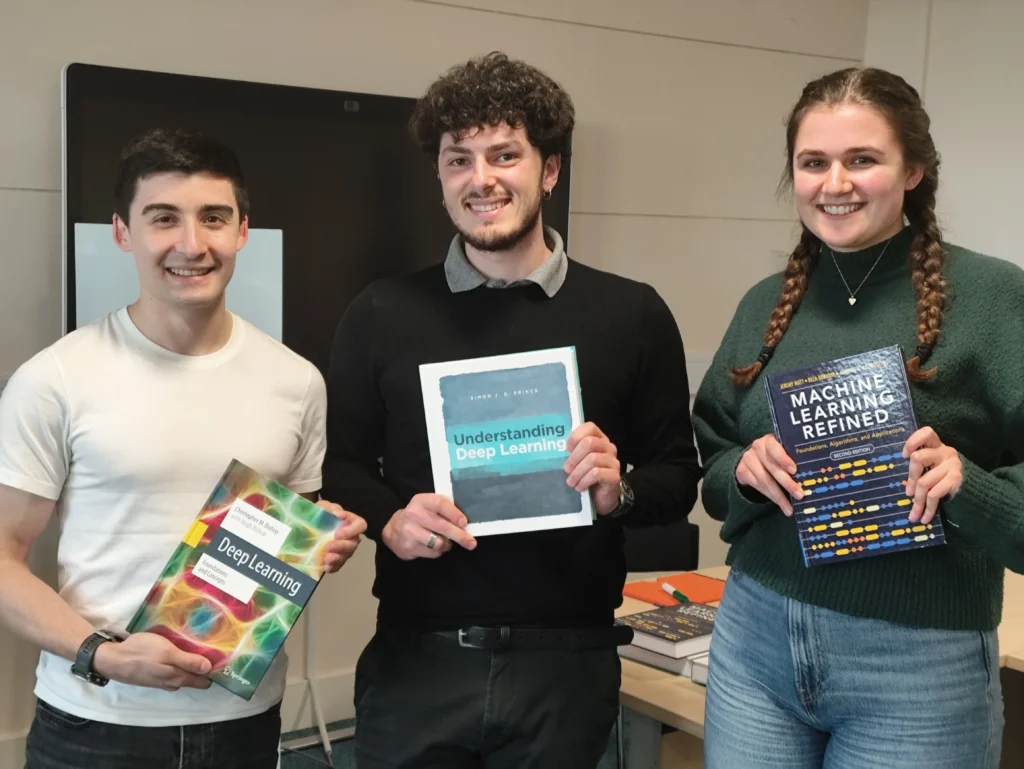
Here’s just some of the feedback from students who attended this year’s event:
What I thought I was great about the school; Overall toolset of ML techniques (knowing where to look for when necessary), concepts and ideas behind ML techniques, tutorials (ready to go, with comments), computational resources given, opportunity to talk to lecturers and other researchers directly, networking.
I generally liked that the course covered a broad range of topics rather than going into details in specific areas; I think it was a good way to understand what options there even are when applying machine learning to scientific research.
MLIPS and finetuning MACE was great. Explanation of GNNs also very good, in general I thought the school was great!
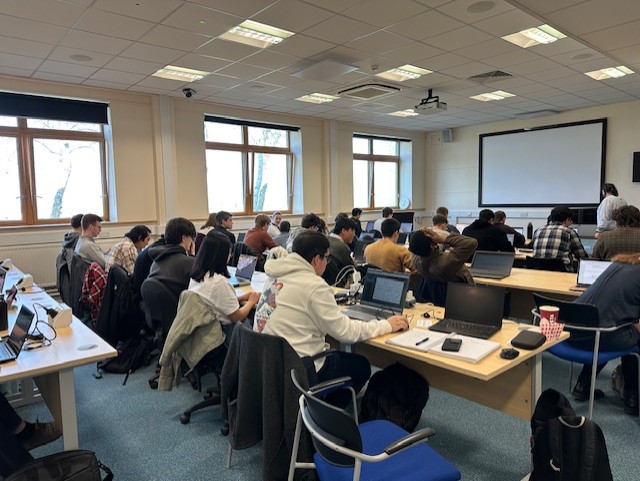
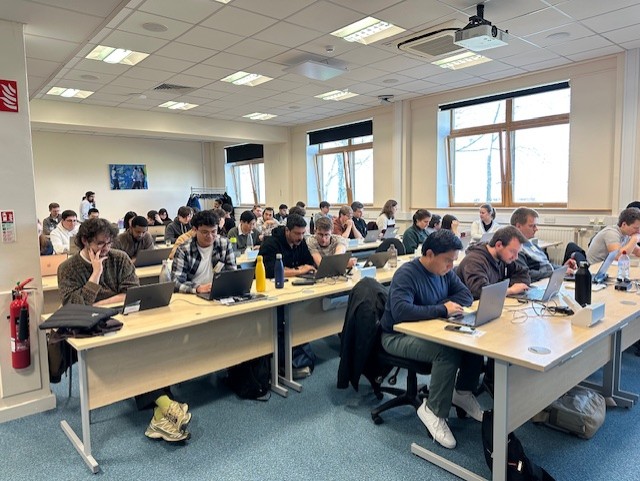
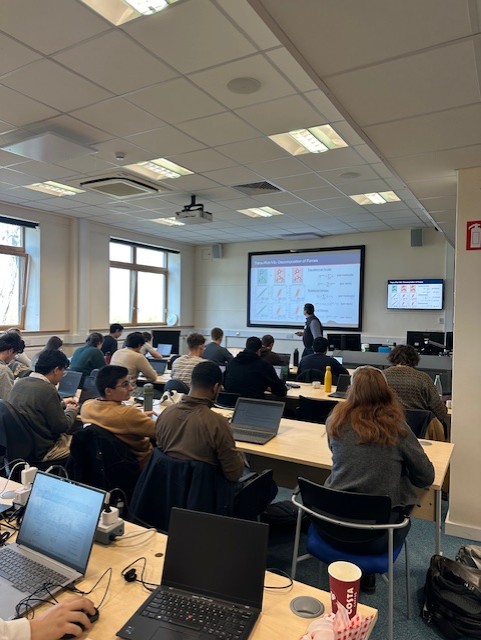
For more information on the school, take a look at the CaMML website
Due to the success of this year’s workshop, we’re already in the planning stages for the 2026 edition — so stay tuned for updates!